Ludo Theunissen, President of the Instituut voor Kredietmanagement
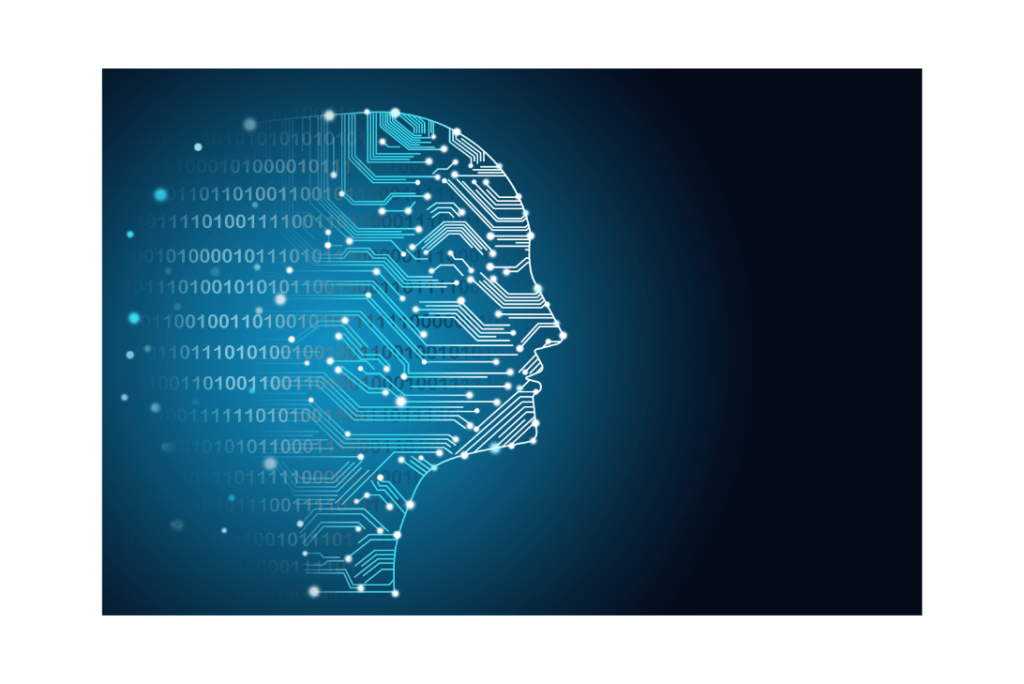
Managing unpaid receivables is more than ever a major challenge for any business. This challenge is today exacerbated by global economic uncertainty resulting from high inflation, the energy crisis, the war in Ukraine, supply chain disruptions … Circumstances that can have a significant financial impact for many companies, forcing even the best payers to defer payments.
And delayed payments by customers have an obvious negative impact on the company’s liquidity.
So ensuring positive cash flow is definitely one of the priorities to manage risk and the impact of unexpected events in these turbulent times.
It is best not to wait for invoices to go unpaid, reducing cash flow.
According to Edouard Beauvois, co-founder and CEO of AiVidens, the problem is clear: “Many companies only deal with their outstanding receivables once the invoice is past due. This is a defensive strategy where the problem is only addressed after the fact. Collectors have less control and recovery becomes more complicated and costly as time passes.”
That means one needs a well-crafted strategy that detects the emergence of problems and their solution as early and accurately as possible.
For this and for optimizing the collection strategy, prediction models can be used.
Mitigate the impact of crisis through prediction
Understanding when and how outstanding invoices will be paid in the future represents a significant competitive advantage for any organization. With accurate and reliable insights into customer payment behavior, cash flow comes back under control by dynamically generating collection solutions tailored to each of its customers.
Take the case of loyal and punctual customers in paying their invoices.
Suppose they face payment problems due to current circumstances. By using advanced prediction systems, the collection strategy can be adapted to the specific context and needs of each customer.
For example, payment deadlines can be extended for some customers. For others, payments can be staggered over a period of time.
This ensures the continuous collection of receivables and also avoids the potential loss of important customers.
The importance of good cash flow planning is clear, but then the next question logically follows: “How can I perfect the forecasts and ensure that my business continues to perform well despite payment risk? And how can I do this while maintaining good relationships with my customers?”
In this volatile environment, the limitations of traditional collection methods that rely solely on historical data and rules predetermined by human operators and only re-evaluated from time to time become apparent.
Therefore, the answer may lie in the use of artificial intelligence.
Optimize your accounts receivable management with artificial intelligence
How can AI be helpful here?
Once again, we turn to AiVidens who has developed an application for this purpose.
The starting point is of course the availability of large amounts of data. Preferably as much as possible and going back a number of years, 3 to 5 years is usual. It is important to use and combine as many sources of data as possible. So not only the obvious data of each customer from the existing relationship, but also external data are included. And this includes data on individual companies as well as economic, sectoral, geographic, social, ….. data.
Daily updates and additions are necessary to keep the dataset as qualitative as possible.
The system will use the dataset by combining 3 different techniques. In addition to classical analysis, an advanced statistical process will be added. And in the process, everything will be analyzed and processed with AI techniques.
That leads to the classification of customers.
This means a payment behavior is calculated for each customer or customer segment. Here it is not only a matter of analyzing and understanding the customer’s historical payment behavior, it is equally – perhaps even more – important to thoroughly analyse the changes that occur and the various influencing factors that are important in this process. It is at this stage that the use of AI techniques becomes primordial. The huge volumes and diverse nature of the data become too vast and complex to be processed with traditional analytical and statistical systems.
So changes in payment behavior are especially important. Indeed, as long as a customer always shows the same payment behavior there is no problem. It does become critical if a customer should change their payment behavior. And when such a thing might happen is just what can be better calculated using AI rather than traditional techniques.
The system can therefore generate alerts based on risk and payment profile, both of which are determined from the extensive and diverse set of data the system uses.
But there is more. For example, the application can determine sensitivity by customer group. Or simulate the effects of different collection strategies.
Fundamentally of course, the system is fed new data daily.
Indeed, the AI application includes a learning process, allowing it to continuously optimize.
AI thus becomes an important tool for credit management and treasury management. The program complements existing financial analyses by using external and predictive data.
The goal of AI is not to replace collection specialists but rather to help them focus on the actions with the greatest impact, identifying risks and the most efficient actions to take.
With these more precise analyses, the picture of how the customer portfolio evolves over time also becomes clearer.
For example, by segmenting risk levels by customer and activity, potential problems can be identified before they arise.
Although AI is sometimes seen as a threat, it is a valuable asset that allows the credit manager to anticipate liquidity problems in order to maintain positive cash flow, and to make the work of the entire team even more efficient.